Generative AI vs extractive AI in legal technology
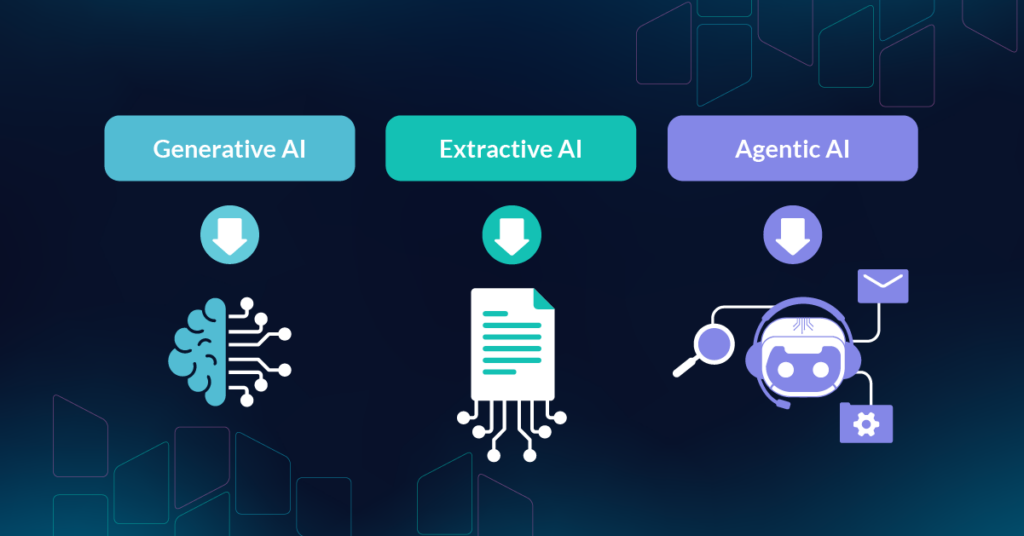
Artificial intelligence (AI) continues to transform the legal sector, continuously offering new ways to enhance efficiency and decision-making for in-house legal teams. But when your goal is simply to understand how to extract value quickly from AI tools, the growing complexity of this landscape can feel prohibitive, prompting more questions than answers.
As you begin to survey the AI-powered legal tech market, you’ll notice the terms ‘generative AI’, ‘extractive AI’ and possibly even ‘agentic AI’ appear on repeat. What do they mean in layman’s terms? And what’s the difference between them in the context of in-house legals’ day-to-day remit?
The good news is that, for the purpose of in-house legal work, understanding the basics of AI in legal technology is simpler than it might seem.
Understanding generative AI
Built on Large Language Models (LLMs), generative AI (GenAI) creates new, human-like outputs based on its training data. This ability to respond dynamically makes it a powerful tool for problem-solving and drafting novel material.
How GenAI works: GenAI predicts the most likely response to a query using its training data. Its applications span far beyond the legal sector; ChatGPT, for example, has gained massive popularity due to its ability to generate text, code and even creative material.
The basics of extractive AI
Extractive AI may not prompt quite the same headlines as its generative counterpart, but its value to in-house legal teams is undeniable. Unlike GenAI, extractive AI doesn’t generate original material. Instead, it identifies and extracts precise details and actionable insights directly from datasets it is trained on.
How extractive AI works: Extractive AI can analyze high volumes of structured and unstructured data, matching patterns found within datasets to deliver specific, referenceable results.
Gen AI vs extractive AI use cases and considerations for in-house legal teams
Now that we’ve covered the basics of GenAI and extractive AI, let’s compare these considerations against common use cases for in-house legal teams.
1. Data scope and reliability
Generative AI acts like a “closed-book exam”, generating new outputs by predicting likely responses. This means it can occasionally invent information, meaning that it requires constant validation. That’s why use cases for in-house legal teams are likely to include the drafting of materials such as templates, legal memos and contracts from scratch.
Extractive AI on the other hand is akin to an “open-book exam”, providing precise outputs only from its trained or uploaded datasets, ensuring verifiable results. For in-house legal teams this could be applied when reviewing existing compliance policy documents or identifying clauses within contracts or pulling data from invoices to populate databases.
2. Risk of hallucinations
Generative AI is prone to hallucinations (producing false but plausible-sounding outputs) because GenAI models prioritize pattern recognition and predict likely outputs, rather than surfacing data which exists in its native format. While this can lead to innovative outputs, it also poses risks in legal contexts requiring factual accuracy. That’s why GenAI is best applied to perform contract data extraction, invoice data extraction, contract redlining, and even eDiscovery.
Extractive AI offers grounded outputs. This means it excels at sourcing and summarizing relevant information, rather than generating new material; because each output ties back to its source data, it’s inherently more reliable. Extractive AI features are also more likely to provide users information to the data source, which means you can conduct due diligence and verify it quickly if this is a non-negotiable.
3. Ethics and compliance
Generative AI has come under scrutiny for potential copyright issues, as its algorithms are often trained on publicly available works without proper attribution. That’s why legal teams should prioritize compliance, privacy and originality when utilizing this technology.
Extractive AI on the other hand, avoids copyright and plagiarism concerns by referencing exact text and providing citations. This traceability is especially crucial for high-stake corporate environments with, for example, tasks requiring direct citations of regulatory documents.
4. Efficiency across legal workflows
Both tools undeniably improve productivity, but the application of each largely depends on the task. For example, GenAI can be valuable for speeding up the creation of material or answering key questions about a document. On the other hand, extractive AI excels in handling repetitive, document-intensive tasks that require precision and meticulous attention to detail, such as extracting renewal dates from a contract.
It’s important to note the distinct user experiences between the two, as these differences can also impact workflow efficiency. For example, GenAI relies heavily on prompts to control and refine its output, requiring more user input, whereas extractive AI presents information with minimal user intervention or refinement needed.
But what about Agentic AI for legal teams?
Step aside GenAI and extractive AI, there’s a new kid on the block (kind of). Agentic AI is gaining recognition as a groundbreaking concept within the context of corporate legal. Unlike extractive or generative AI, agentic AI is designed to pursue specific, dynamic goals and manage complex, multi-step actions that require continuous decision-making and adaptability. The biggest drawcard? Because it’s self-prompting, agentic AI doesn’t require in-house lawyers to become prompt engineers.
Here are a few examples of how agentic AI can be applied within legal teams:
Automated contract generation and review
Agentic AI has enormous potential to streamline contract drafting and review for in-house legal teams by generating customized contracts using templates and clauses, and spotting risks or compliance issues to save time and improve accuracy. This may allow legal counsel to create NDAs or licensing agreements far more quickly while ensuring compliance.
Due diligence
When it comes to conducting due diligence for tasks such as reviewing contracts, extracting key clauses and generating reports, AI is already widely used. However, by leveraging agentic AI, the many other tasks due diligence calls for (which traditionally require human-centric input) could be completed automatically. For example, tasks that require analysis and planning, such as data interpretation, assessing risk potential, team collaboration and decision-making, could all fall within the remit of agentic AI.
As the capacity of agentic AI evolves, the level of human supervision involved in using this technology is expected to decrease. Because it has the ability to proactively manage processes and make decisions to ensure that tasks are completed efficiently, agentic AI carries a lot of potential for further unlocking the strategic impact of in-house legal teams.
AI technology for in-house legal
Now that we’ve unpacked the basics of GenAI, extractive AI and agentic AI, you might be ready to learn more about how to adopt these systems safely and responsibly. To learn more about how to unlock new levels of efficiency, accuracy and innovation with tools like LawVu, click here.
And if you’re still on the fence about where to start with AI or need to do more research, check out our comprehensive AI guide for in-house counsel.